[ad_1]
The “sixth knowledge platform,” or clever knowledge platform, represents a cutting-edge evolution in knowledge administration.
It goals to help clever functions via a complicated system that builds on a basis of a cloud knowledge platform. This basis makes it doable to combine knowledge throughout totally different architectures and programs in a unified, coherent and ruled method. Harmonized knowledge allows functions to make use of superior analytics, together with synthetic intelligence, to tell and automate choices.
However extra importantly, a typical knowledge platform allows functions to synthesize a number of analyses to optimize particular aims, guaranteeing, for instance, that short-term actions are aligned with long-term impacts and vice versa. Greater than only a dashboard that informs choices, this technique more and more incorporates AI to enhance human decision-making or robotically operationalize choices. Furthermore, this intelligence permits for nuanced decisions throughout numerous choices, time frames, and ranges of element that have been beforehand unattainable by people alone.
On this Breaking Evaluation, we introduce you to Blue Yonder, a builder of digital provide chain administration options that we consider represents an rising instance of clever knowledge functions. With us at present to know this idea additional and the way it creates enterprise worth are Blue Yonder Chief Government Duncan Angove and Chief Know-how Officer Salil Joshi.
Key points
Let’s body the dialogue with among the key points we need to deal with at present.
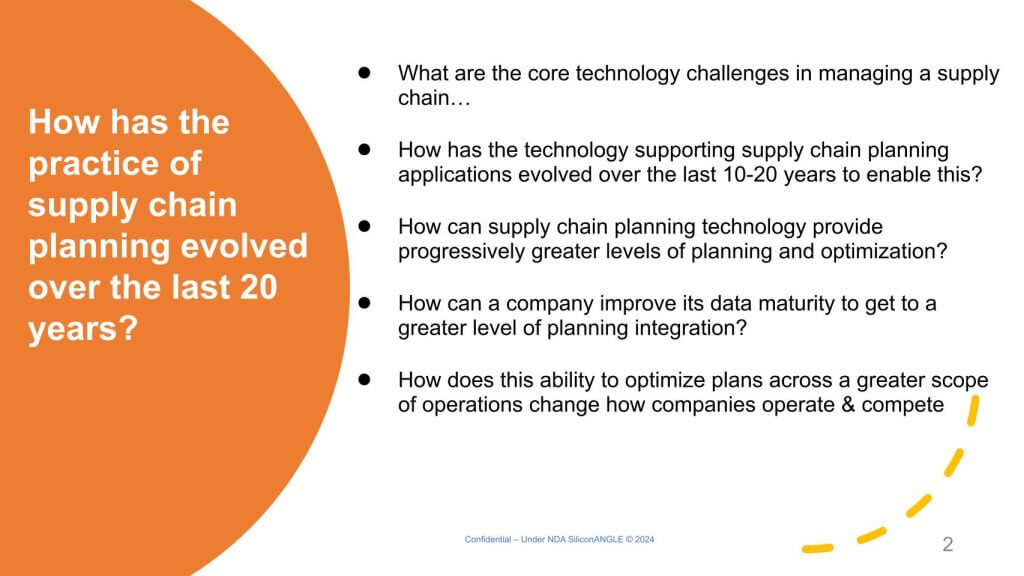
The observe of provide chain planning and administration has advanced dramatically over the previous couple of a long time and hopefully we’re going that can assist you perceive how legacy knowledge silos are breaking down, how AI and elevated computational capabilities are forging new floor, and the applied sciences which might be enabling a lot larger ranges of integration and class in planning and optimization throughout a provide chain ecosystem. We’ll attempt to deal with how organizations can evolve their knowledge maturity to attain a larger degree of planning integration and the way they’ll dramatically enhance the scope of operations and their aggressive posture.
The issue assertion
Beneath is a graphic that we scraped from the Blue Yonder web site. The objective right here is to optimize the way to match provide and demand in keeping with an goal comparable to profitability. To take action, you want visibility on many alternative components of the availability chain. There’s knowledge about buyer demand and totally different components of an inside and exterior ecosystem that decide the power to provide that demand at a given time. The info in these programs traditionally has been siloed, leading to friction throughout the availability chain.
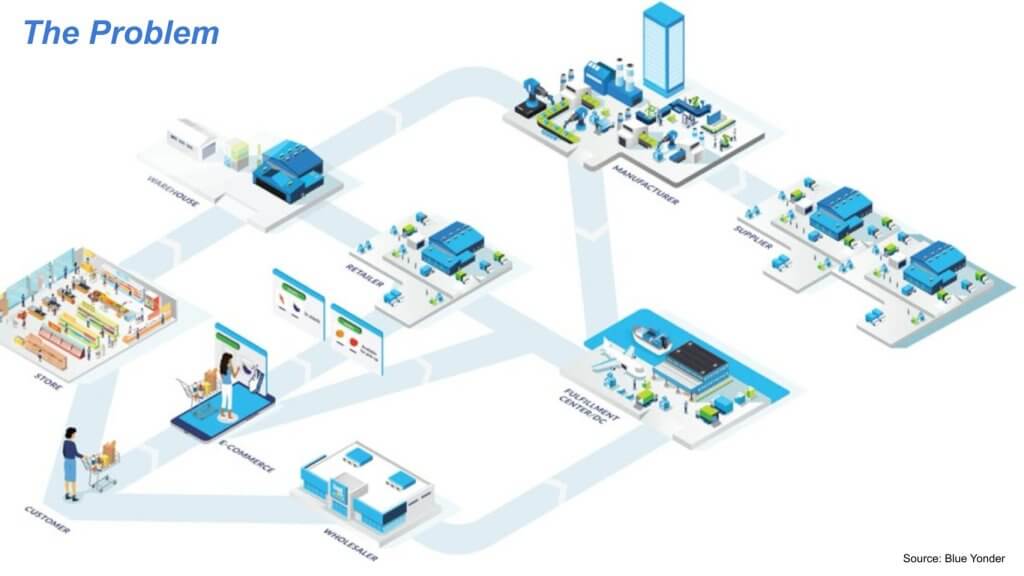
Duncan Angove elaborates:
Provide chain is arguably probably the most complicated and difficult of all of the enterprise software program classes on the market, simply because, by definition, it’s provide chains that includes a number of firms versus one like an ERP or a CRM coping with clients, and simply the information and complexity of what you’re coping with when you consider, in the event you’re a retailer, SKU occasions location occasions day. So it’s billions and billions of information factors. And so, that makes it very, very difficult. So you must predict demand on the shelf, after which you must cascade that each one the best way down the availability chain, from the transportation of the vehicles that get it there to the warehouse, inbound and outbound, again to the distributor, the producer, that producer’s provider’s provider’s suppliers.
So it’s very, very complicated when it comes to the way you orchestrate all of that, and it includes billions and billions of information factors and excessive problem in orchestrating all of that throughout departments and throughout firms. And provide chain administration, in contrast to different classes of enterprise software program, has all the time been very fragmented, to the purpose that Dave made. And meaning it’s fragmented when it comes to the information, the functions, a really numerous software topology, which implies you could have unaligned stakeholders in a provide chain. Due to the amount that’s concerned right here, it’s typically being a really batch-based structure versus real-time, which implies there’s a variety of latency in transferring knowledge round, manipulating it, and all of that.
And it’s typically being restricted by compute, which signifies that, once more, it’s batch-based, and corporations typically commerce out accuracy for time. It simply takes too lengthy due to the compute, and it’s typically been very, very exhausting to run within the cloud, as a result of it’s mission-critical. It requires on the spot response time. In the event you’re attempting to orchestrate a robotic in an aisle in a warehouse, that requires instantaneous decisioning and execution. So it’s very, very difficult from that perspective. And on the finish of the day, it’s essentially a knowledge drawback and a compute drawback. In order that’s how I’d characterize it.
Salil Joshi provides the next technical perspective:
So if you consider provide chain issues and the useful silos that, inherently, provide chain is in, the pretty static nature of how provide chains have been in place, at present, expertise is such that we’re in a position to resolve a a lot bigger drawback than what we used to up to now, the place we’re mainly saying that previously, our skill to unravel issues was restricted by, to level Duncan made, across the compute. We now have hyperscalers like Microsoft, who’re in a position to present us an infinite compute.
Via the Kubernetes clusters that we’ve got, we’re in a position to scale horizontally and resolve the issues that we, frankly, weren’t in a position to resolve up to now 5, 10 years. The entire nature of getting a knowledge cloud now obtainable to us, separating compute in opposition to storage, that permits us to scale in a fashion that’s much more efficient, cost-effective, in addition to skill to be much more real-time than what we have been up to now. Once more, from a knowledge cloud perspective, one of many issues that has all the time bogged provide chain points is integrations.
If you consider the entire totally different programs that we have to have in place from an integration standpoint, knowledge flows from one to a different system. It was extraordinarily complicated. Now, what we’ve got is skill the place we’re in a position to materialize knowledge in a way more seamless method from one facet of the availability chain to the opposite facet, in order that visibility is there. And the power to then scale that past what we had up to now is now evident. So, for instance, we associate with Snowflake, and what we’ve got leveraged there’s this facet of zero-copy clones, which is basically a capability to materialize knowledge, clone knowledge, with out replicating it time and again.
And on account of which, we will play out operational situations, determine what’s going on by altering the levers, after which selecting what’s the most optimum reply that we need to get. So the trendy knowledge cloud helps us resolve issues at a scale that we weren’t in a position to resolve up to now. And eventually, adopting generative AI to actually change the interface of how customers have used provide chains up to now can be an important facet of how we resolve provide chain challenges sooner or later. So these are the assorted totally different expertise advances that we see now that we’re ready to make use of to unravel among the challenges that Duncan talks about, from a compute perspective and from a knowledge integration perspective.
Q. Salil, Duncan had talked in regards to the scope of provide chains now. And whenever you’re speaking throughout firms in an ecosystem, even inside a single firm, all these legacy functions are silos. How do you harmonize the information in order that your planning parts are primarily analyzing knowledge the place the that means is all the identical?
Salil’s response:
So it begins with the information. Historically, in provide chain, knowledge has been locked inside software silos. And that has to come back collectively meaningfully in what we name a single model of the reality, have a single knowledge mannequin that’s constant throughout the complete enterprise, bringing the canonical knowledge type in place such that we’re in a position to outline the entire atomic knowledge and retailer the atomic knowledge in a single place, in the suitable knowledge warehouse. On high of that, then you must set up or have what we’re calling the logical knowledge mannequin or the semantic knowledge mannequin, in some sense, the enterprise data graph, the place you could have all of the definitions of the availability chain for that individual enterprise outlined in a really, very constant method, such that you’ve got all of the constraints outlined in the suitable method. You have got all of the aims which might be linked in the suitable method, and the entire semantic, logical data that’s wanted for fixing the availability chain issues constantly can be found. So after getting the information in a single model of fact, after which this logical knowledge mannequin, you’ll be able to then have functions join to one another in a significant method the place not solely the information, however the context can be handed in a related method.
Q. Simply to make clear, the data graph, is that applied inside Snowflake as a knowledge modeling train versus one other expertise that’s nearly like a metadata layer exterior the information cloud?
It positively makes use of Snowflake, however there’s a expertise that we’ve got used about Snowflake, on high of Snowflake. There are a few methods. We’ve got homegrown expertise, but additionally leverage expertise from RAI (RelationalAI) that builds the data graphs to permit us to unravel the bigger, complicated optimization issues.
Our dialogue additional uncovers that together with Blue Yonder IP, this all runs as a coprocessor natively inside Snowflake for these particular workloads. Based on Duncan Angove, it’s significantly good at solvers and AI. Nevertheless it runs in a container in Snowpark and is native to Snowflake.
Addressing the issue
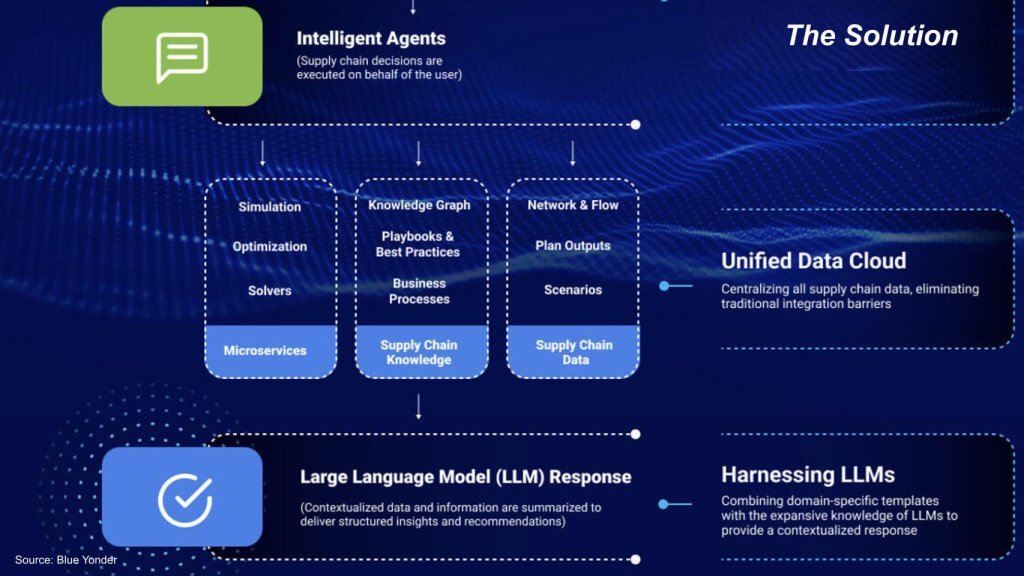
We pulled the above conceptual framework from Blue Yonder’s web site. It mainly describes the corporate’s expertise stack. Our take is the method is to achieve visibility inside all of the components of the availability chain, however to unravel the issue it takes an end-to-end programs view. After you have visibility, you’ll be able to harmonize the information, you may make it coherent, after which you are able to do evaluation on not solely the person components, however how they match into the system as a complete, and the way all these views inform one another. And the purpose is, that is an built-in evaluation with a unified view of the information. You’ve bought domain-specific data, and you then contextualize that knowledge, not simply from a person part standpoint, however the complete system.
Q. Please clarify how you consider the Blue Yonder stack and the way you consider fixing this drawback.
Salil responds:
As I discussed, the very first thing is to have the information in a single model of the reality, and set up that in place, have the logical knowledge mannequin outlined in a really dynamic method. So these are the 2 first constructing blocks of the piece. We additionally then have what we name an occasion bus, due to the truth that, Duncan talked about this, that offer chains have been historically in a batch mode. And now, with the requirement for real- time responses, with the requirement for event-driven responses, we’ve got an occasion bus on which the entire provide chain occasions get carried via, and these occasions are then subscribed to by the assorted microservices that we play out throughout the complete spectrum of our provide chain.
So what’s going to find yourself occurring is, as an occasion is coming via, whether or not or not it’s a requirement sign, whether or not or not it’s provide disruptions which might be occurring, these occasions are then picked up by the assorted microservices on the planning aspect of the equation or the execution aspect of the equation, such that the suitable responses are supplied. And never solely that, as soon as the occasion is handled, understood, reacted upon, then alerts and actions are despatched to downstream processes, the place the downstream processes then take that under consideration after which take it out into actioning and execution. In order that’s the general view that we’re setting up collectively the place, one, you could have the information, the logical knowledge skill to absorb occasions, perceive what that occasion does from an insights perspective, create the actions which might be required for downstream processes, and be sure that these actions are executed in opposition to. As soon as these actions are executed in opposition to, you get a suggestions loop into your total cycle such that you’ve got steady studying occurring via the method.
Q. You had talked about brokers earlier. A few of what we’re studying about is that brokers, the best way we’ve seen them referred to up to now, are clever person brokers the place they’re form of augmenting the person interface. However we’re listening to from some folks as nicely that among the software logic itself is being realized in these brokers, the place we’re used to having deterministic software logic specified by a programmer. However now, the brokers are studying the foundations, however probabilistically. Are you able to clarify the excellence between an clever agent that augments a person a dashboard and an agent that really is taking accountability for a course of?
Salil’s response:
I’ll provide you with an instance within the forecasting world. Previously, we’d forecast based mostly on historic knowledge after which say, “Here’s a deterministic calculation of what the forecast will appear like from the historical past,” and also you get a single quantity for it. And let’s say the reply is X quantity at a selected retailer at a selected time. Now, within the new world, you get a number of alerts, that are causals in nature. Proper? There are some that are inherent throughout the enterprise, some which might be market-driven.
And you must sort of get a way of, what are the chance of these causals to occur? And on account of that chance, what’s the vary during which an end result will happen? So, for instance, as an alternative of getting one quantity for a forecast, you now have a chance curve on which this forecast is on the market to you, the place, relying on the totally different causals which might be on the market, you should have a special end result. And what’s going to find yourself occurring is, when you could have that data over a time period, a system will study the way during which these chance curves are taking impact in reality, and that these learnings will, once more, make the system way more clever than what it’s at present. And on account of which, you begin getting increasingly more correct solutions because the system learns.
Duncan provides:
It’s an excellent query as a result of essentially, prediction and provide chain is the place the sphere of operations analysis began…bear in mind the milk run, the beer run solvers, which aren’t attractive anymore, as a result of now gen AI is what’s attractive, and you then had deep studying, machine studying utilized to it, and now there’s generative AI. These former issues, to your level, are deterministic. Precision issues. It’s arithmetic. Proper? These are stochastic programs. Hallucination right here is nice. It’s known as creativity and brainstorming, and it will possibly write essays and generate movies for you. That’s dangerous in math world, they usually’re not good at math at present. Nonetheless, they’re going to get higher and higher and higher. So once we take into consideration intelligence, it’s the mix of all of these items. We’ve bought solvers that do an exceptional job, for instance, within the semiconductor trade, ran the desk final yr, as a result of it does an excellent job of that.
We’ve bought machine studying. We run 10 billion machine studying predictions a day within the cloud for recent meals, as a result of it’s very, excellent at that. Proper? And you then’ve bought generative AI, which is nice at inference and creativity and problem-solving. The mixture of these three issues we form of check with because the cognitive cortex. Proper? It’s the mix of these three issues, which supplies you a brilliant highly effective end result. After which there’s tactical issues, just like the token size of the sphere. It might be insane if we use Gen AI for forecasting. What are we going to do? Feed 265 billion knowledge factors into the immediate field? No. So what occurs is, as Salil was speaking about this event-driven structure, as occasions occur, that’s a immediate. It goes right into a immediate, and Gen AI is aware of to name this solver to do that. And when it figures out what it ought to be doing, it calls an execution API, “Transfer a robotic in a warehouse to select extra of this merchandise. ” Proper? That’s form of the way it hangs collectively.
And Gen AI is the orchestrator, that’s what we name it, the Blue Yonder Orchestrator.
Q. Because the brokers get higher, or as the inspiration fashions get higher, at planning execution, do you see them taking on accountability for processes that you just’ve written out in procedural code, or do you see them form of taking up workflows that you just couldn’t have expressed procedurally as a result of there are too many edge circumstances?
Duncan responds:
I believe it’s a little bit of each. So initially, individuals are attempting to make use of gen AI to unravel issues which have already been well-solved by different expertise, whether or not it’s ML or it’s a solver or it’s RPA, which isn’t attractive anymore both, or enterprise search. Let’s use it for what it’s good at and perceive the deficiencies it has proper now. And once more, it’ll get higher and higher. Our perception is, is that issues that may be realized and are closely language- or document-oriented, are ripe for this. That’s why it’s gone after legislation and all of this different stuff. Proper? It’s simple to do this. Hear, provide chains run on paperwork, as you already know, buy orders, invoice of ladings. Proper? It’s all that EDI stuff that we used to dread. These are all paperwork. So there are issues there that can be simpler to do.
Our perception is that workflow functions the place it’s very forms-based are at excessive danger of being displaced for certain. Planning is way, a lot tougher. Nobody desires to take a look at a time horizon pivot desk via a chatbot. I imply, nobody desires a chatbot on high of their Excel. You may want some generative AI embedded in cells and stuff like that, however these can be tougher to go after, they usually do require the mental property we’ve got round solvers and ML and all of that. However finally, we’re transport brokers that amplify all of the totally different end-user roles that we’ve got. However a few of them will go an extended, lengthy solution to lowering the quantity of labor they’ve carried out, repetitive stuff. There are some roles, like tremendous refined planners in semiconductor, the place it’s tougher, however there’s nonetheless progress you may make there. However on the finish of the day, these items are simply going to get smarter and smarter. I imply, let’s be clear. The big language fashions at present don’t even study. Reminiscence is a brand new factor. So that you want to suppose that the longer an finish person interacts with their very own agent, it learns their model of working, it remembers context, and it simply will get higher and higher and higher and extra helpful over time. And it’ll occur rather a lot quicker than we predict.
The connection between digital and bodily worlds
In a considerably tangential dialog we mentioned the the linkages between the digital and bodily worlds, the connection to the sixth knowledge platform and the elemental “plumbing” that’s been laid down over time – in robotic course of automation, for instance – and naturally in provide chain. The next summarizes that dialog:
Gen AI is a risk to legacy RPA programs as proven within the Enterprise Know-how Analysis knowledge. In the course of the COVID-19 pandemic, RPA noticed a major surge above conventional momentum strains. Nonetheless, with the arrival of huge language fashions, the keenness for standalone RPA options has waned. Firms that haven’t transitioned to extra complete, end-to-end automation imaginative and prescient are starting to see the restrictions of their approaches.
The significance of connecting digital capabilities to bodily world functions can’t be overstated. Efficient automation programs don’t simply function in a digital vacuum; they combine deeply with bodily programs, permitting for tangible actions like rerouting site visitors or managing logistics. The foundational work carried out by RPA applied sciences, laying down the digital plumbing, is crucial because it allows this bridge between the digital and the bodily worlds. The identical is true in provide chain.
For instance:
-
- Execution programs: These programs, like warehouse administration or transportation programs, play an important function in translating digital orders into bodily actions.
-
- API integration: The event of software programming interfaces has facilitated actions within the bodily world, comparable to transferring vehicles, altering routes, and managing product deliveries.
That is why we’ve been wanting to speak to Blue Yonder within the context of the “sixth knowledge platform,” which we’ve described because the “Uber for everyone.” This platform goals to contextualize folks, locations and issues — components generally understood by the general public (i.e. issues), however usually summary in database phrases (i.e. strings). The problem and alternative lie in reworking these summary knowledge strings into actionable real-world entities.
In sensible phrases, this includes:
-
- Integration of analytics: Merging analytics throughout numerous functions to offer a unified view that permits totally different programs to tell and improve one another’s capabilities.
-
- Provide chain as a bridge: Specializing in the availability chain as a crucial connector between the digital knowledge and bodily execution, highlighting the technical complexity and necessity of integrating these domains for efficient operations.
We see this holistic method to digital-physical integration as essential for the subsequent technology of clever knowledge functions, guaranteeing that technological developments translate into efficient, real-world outcomes.
Digging deeper into the Blue Yonder method
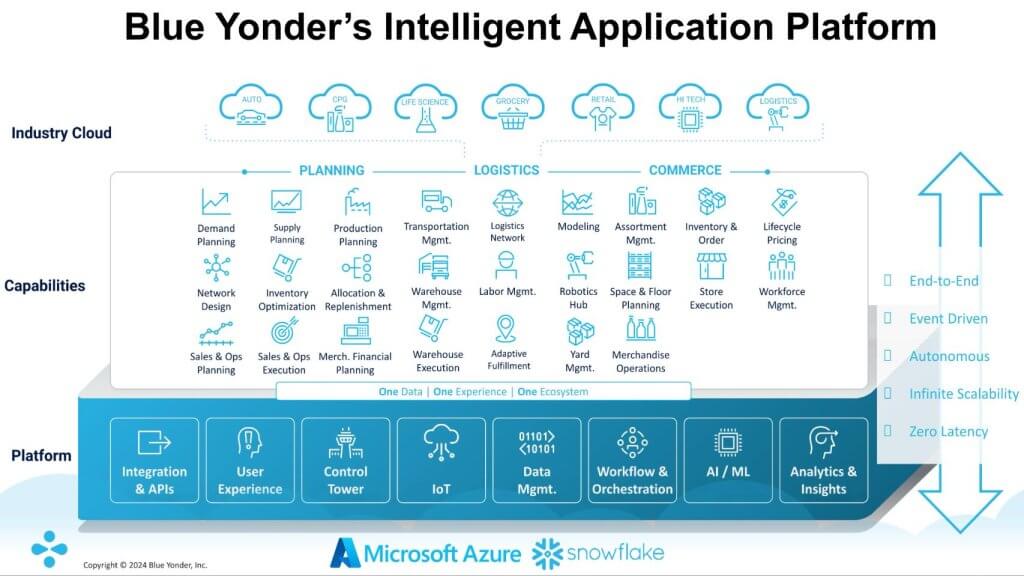
The graphic above depicts the assorted components of the Blue Yonder answer. We needed to raised perceive the technical components of this image. We requested Blue Yonder to clarify how the functions it delivers work collectively utilizing that frequent view of information that we’ve been speaking about. How do you combine all of the analytics such that the functions which have totally different particulars can inform one another?
Duncan frames the dialog as follows:
I’ll tee up the design, what the design aims have been, and the way what’s occurring on this planet formed it, and Salil can carry it to life with the appliance and tech footprint and a few examples.
So nobody knew what a provide chain was earlier than COVID. It’s humorous, when one thing breaks, folks abruptly acknowledge there was one thing doing one thing. However actuality is, we’re going via a generational shift in provide chains we haven’t seen in 30 years or 40 years. For the final a long time, it’s been about globalization and productiveness, and let’s use China because the world’s manufacturing unit after which ship stuff in every single place. And the philosophy was throughout simply in time. OK? These two issues sort of did it. And there’s a lot disruption occurring now, the rewiring from China, the re-shoring of producing, the friend-sourcing. There’s sustainability. There’s e-commerce. There’s demographic time bombs with labor shortages. There’s the impression of local weather change, the Panama Canal, the Mississippi River, international instability, the Houthis, you title it.
We’ve by no means seen it like this. So J.P. Morgan did a report of 1,500 firms within the S&P, and within the final two years, working capital has elevated by 40%. That’s stock. It’s staggering, proper? That’s cash that isn’t being invested in progress or within the client. It’s sitting on somebody’s steadiness sheet. That may’t be the reply to resiliency. The reply is software program, and reimagining how a provide chain ought to run with software program. And what firms want is agility. They want the power to aggressively reply to danger and alternative, they usually should be absolutely optimized. So the issues that you just want technically there’s you want visibility. It’s exhausting to be agile if one thing hits you on the final minute. You want to have the ability to see there’s a provide disruption. As we speak, it takes, on common, four- and-a-half days for an organization to study of a disruption in provide.
That doesn’t work. You should use prediction in AI. I can predict that I’ll have a provide situation, that this order goes to be late, that this spike in demand goes to be occurring. So agility is crucial, and it’s fueled by expertise. The second factor is, how do you aggressively reply? It’s very exhausting in provide chain to do this, as a result of everybody’s siloed. You want everybody coordinated to go resolve an issue. The logistics folks which might be scheduling vehicles want to speak to the folks within the warehouse, within the retailer, the folks which might be determining what to purchase. That has to function as one, and it doesn’t at present as a result of it’s in silos, and all the information is fragmented and in every single place. In order that’s the second factor. After which to be absolutely optimized throughout all of that, you want infinite compute and also you want unified knowledge, and you must get out of batch, and you must join the decisioning programs, which is planning, to the execution programs.
These are the issues that you just want. That design level truly describes an clever knowledge app. That’s precisely what solves this. In order that’s a little bit bit on the design level we intention for.
Q. Salil, give us an instance of a use case, of a provide chain the place there’s an unanticipated disruption and you must replan so that you just’re altering each your long-term plans and your short-term plans, and also you need to harmonize all these issues, after which drive execution programs with a brand new set of plans.
So take, for instance, a big order has simply come into your laps. You had a plan in place. That plan had been despatched to your warehouses, the logistics programs, and all of that’s in place and it’s in movement. And a big order that has are available and preempted, proper? This preemptive order now has are available, is, one, there’s an order reprioritization that has to occur. As soon as that order reprioritization has occurred, it has to then go into your execution programs the place now, abruptly, the warehouses have to concentrate on the truth that, “Oh, now my choose cycles have to vary. My transport has to vary. The logistics plan that was in place and was in execution has to vary. ”
And at present, as Duncan talked about, these alerts usually are not in actual time. These alerts occur in a batch setting. So what finally ends up occurring is you aren’t ready to reply to this massive order that has are available. So what we’ve got began placing collectively is that this interoperability, as we name it, or the connection throughout the assorted totally different programs the place… And all the best way from the planning aspect of the equation to the execution aspect of the equation, there’s a connection.
Now, the interoperability, on this most simple type, could possibly be simply knowledge integration, proper? However that’s not adequate. The info integration and the extent of evolution is to say, “Now I’ve workflows that I’ve established which might be in a position to join the totally different adjustments that.… The beginning level of the disruption and the entire penalties downstream into the system are linked by a workflow foundation.” The ultimate evolution of that’s that I’m in a position to orchestrate the entire items of the puzzle that I’ve throughout the provide chain collectively, and optimize it collectively. So that’s actually the nirvana that you just draw into.
Duncan provides a number of key factors together with a give attention to sustainability:
Only a actually easy instance. Firms spend a lot time and mind on the plan. It’s most likely the neatest folks. That’s the place the AI is. There’s all this manipulation to get it there, and it doesn’t survive the day. You launch it out, go choose these orders. We’ve forecasted demand on the retailer, all of that. And what you already know is a robotic goes down an aisle, and by the best way, we’ve not simply deliberate the right order, we’ve optimized it in transportation. The load is full. The entire thing is gorgeous. Proper? A robotic goes down an aisle, and the stock’s broken for one of many gadgets that need to go on the truck. And what occurs at present is that truck goes out with out these orders on it and we short-ship a retailer, as a result of there’s no closed-loop suggestions, there’s no occasion, something. Proper? What occurs sooner or later world is the robotic goes down there.
It instantly communicates that order, “Merchandise quantity seven is just not obtainable. ” It calls an order sourcing engine, figures out it will possibly choose up from one other supply, communicates again to the planning system. The planning system says, “Put this order on that truck as an alternative. ” It calls load constructing, re-optimizes the load, and that truck goes out full. Full, and the order is happy. All of that occurs in seconds whenever you join all of these items in actual time and you’ve got infinite intelligence. By the best way, it’s additionally a extra sustainable order. You despatched out a less-than- full truckload within the earlier instance, so that is additionally extra sustainable. And that’s saying: Clearly, we introduced the signing of 1 community final month, which takes that very same worth proposition throughout a multi-tier community. So the identical instance might have been the place, as an alternative of the stock being broken in an aisle, it could possibly be a provider speaking to you, “Hey, we will’t fulfill this order.”
You robotically discover an alternate provider. It calls the service. The service received’t tender the load, until it is aware of that the dock door obtainable, calls the dock door system, “Sure, the door’s obtainable. Sure.” And the entire thing is simply orchestrated. So planning turns into a real-time decisioning engine. The entire thing is event-driven. So it’s extra sustainable. It’s extra environment friendly. It’s extra resilient.
The most effective software use case for the Clever Information Platform
In our view, that is the perfect software use case that we’ve had for the clever knowledge platform, which is, when you’ve bought this knowledge harmonized from all these totally different sources, even exterior the boundary of an organization, you then harmonize the that means. The expertise appears to be a data graph. It’s not clear to us, truly, how else you are able to do it aside from embedding the logic in a bunch of information transformation pipelines, which isn’t actually shareable and reusable. However then when you’ve bought that knowledge harmonized, you could have visibility, after which you’ll be able to put analytics on high. Nevertheless it’s not one analytic engine. It’s many analytics harmonizing totally different factors of view. After which, whether or not it’s via RPA or transactional connectors, that’s the way you then drive operations in legacy programs. That’s the connection between the clever knowledge platform driving outcomes within the bodily world.
Q. Is {that a} truthful recap of the way you guys are fascinated by fixing this drawback?
Duncan responds with a view of how this adjustments enterprise software program deployments:
That’s proper. And there’s truly one other aspect to this. It additionally adjustments the best way enterprise software program is deployed. So the best way you’d usually deploy a planning system is you must go and use ETL from all these disparate databases and functions. You batch-load it into the plan, and you then do a bunch of planning, and all of that. Within the new world, I imply, simply say half our clients no less than use SAP. Most of that’s the planning knowledge that we want. They’ve already bought it in Snowflake. We go to the shopper and we are saying, “Are you able to give us learn entry to those fields?” We don’t transfer the information. We run the clever knowledge app natively on it. There’s no migration. There’s no integration. There’s nothing. The data graph supplies context and permits us to know what it’s. “Oh, wait a second. We need to seize some climate causals.” Snowflake Market. The info is already within the unified cloud. We’re not transferring something. We’re simply doing a desk be part of.
“Oh, the CPG firm want to see what that gross has deliberate.” You’ve bought your CPG knowledge as: Hey, there’s a be part of between the CPG knowledge and our knowledge and the causal knowledge, and we’d run our forecasting engine on high of it. It’s game-changing. You progress the functions and the method to the information. You don’t do the other. So the velocity at which you progress is dramatic. We’ve deployed all of our mental property as microservices on the platform. It’s a forecast as a service. It’s a load construct as a service. It’s slotting optimization within the warehouse. So these are all clever knowledge apps sitting on unified knowledge.
Clever knowledge apps are coming: some remaining ideas
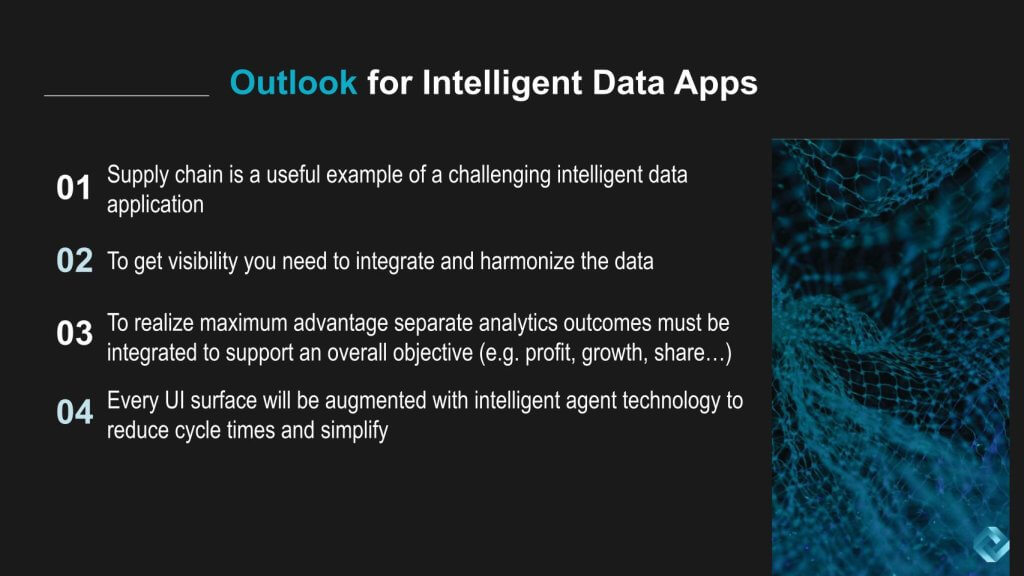
We’ve chosen provide chain as an amazing instance of the sixth knowledge platform or the clever knowledge platform. As we heard from Blue Yonder, provide chain is a superb instance as a result of it’s difficult and there are such a lot of transferring components. There’s a variety of legacy technical debt that must be abstracted away.
To make the most of these improvements, you must get your knowledge home so as. Blue Yonder and Snowflake’s prescription is to place all knowledge within the Information Cloud on Snowflake. This can be a matter for one more day, however there are headwinds we see out there in doing so. Many purchasers are selecting to do knowledge engineering work exterior of Snowflake to chop prices. They’re more and more utilizing open desk codecs comparable to Iceberg, which Snowflake is integrating. They need totally different knowledge sorts together with transaction knowledge and Snowflake’s Unistore continues to be aspirational.
Furthermore, many purchasers we’ve talked to need to preserve their Gen AI actions on-premises and convey AI to their sovereign knowledge. They might not be prepared to maneuver it into the Information Cloud. However Snowflake and Blue Yonder seem to have a solution in the event you’re open to transferring knowledge into Snowflake. The enterprise case on this complicated instance might very nicely justify such a transfer.
As soon as your knowledge home is so as, you’ll be able to combine the information, make totally different knowledge components coherent, i. e., harmonize the information. And we’re taking a system view right here. Oftentimes, managers are going to possibly do an amazing job of addressing a bottleneck or optimizing their piece of the puzzle. And, for instance, they could dramatically enhance their output, solely to overwhelm another a part of the programs and convey the entire thing to its knees, primarily simply transferring the deck chairs round, or possibly worse.
And eventually, with gen AI, each person interface floor goes to be enhanced with an agent expertise. It’s going to cut back cycle occasions over time, dramatically simplify provide chain administration. And on this instance, sure, provide chain, but additionally all enterprise functions. And a really highly effective takeaway from at present is: The reply to enterprise resilience is just not extra stock, it’s higher software program. It’s the intelligence knowledge platform.
Salil’s remaining ideas:
I want to say, with the provision of expertise now on our fingers, whether or not or not it’s ML/AI, whether or not or not it’s the infinite compute, whether or not or not it’s the platform knowledge cloud, whether or not or not it’s now generative AI, this complete notion of the tip to finish, the entire notion of the linked provide chain, the power to have that visibility, skill to orchestrate throughout the complete spectrum… firms will now be capable to begin measuring, at a really totally different degree, the aims and the metrics relative to what they’ve carried out up to now. Now not are you going to speak about price per case at a warehouse.
You’re going to speak about price to serve at a buyer degree. So it’s going to be very totally different transferring ahead with these applied sciences at play. With the sort of superpowers that these programs are going to offer, the software program goes to offer, the important provide chain operator function goes to vary drastically. Our provide chain operators will turn out to be superheroes. The notion of, you touched upon this, round sustainability and ESG. There’s not going to be simply metrics and measures which might be stories down within the dungeons, however it’s actually going to be a part of the optimization resolve, and get higher solutions to this. So firms will turn out to be that rather more aggressive due to the truth that we’ve got these provide chain functions in place, and that’s the joy of pivoting to this new transformation.
Duncan’s remaining feedback and takeaways:
The best way to unravel resiliency is just not stock, it’s software program. And that’s precisely what we’ve constructed, next- technology options that permit firms to be extra agile, aggressively responsive, and absolutely optimized, and all in-built what we predict is the next- technology enterprise software program stack with clever knowledge apps. And that’s what we’ve constructed. So we’re dwelling in a second in time the place provide chains have by no means been extra unsure, and transformation is required, identical to we simply lived via the final 20 years of digital transformation, which was form of consumer-driven, after which we’re seeing the identical necessity now round provide chains. So thanks very a lot for having us on. As all the time, actually loved the chat. You guys requested very insightful questions.
Keep up a correspondence
[ad_2]